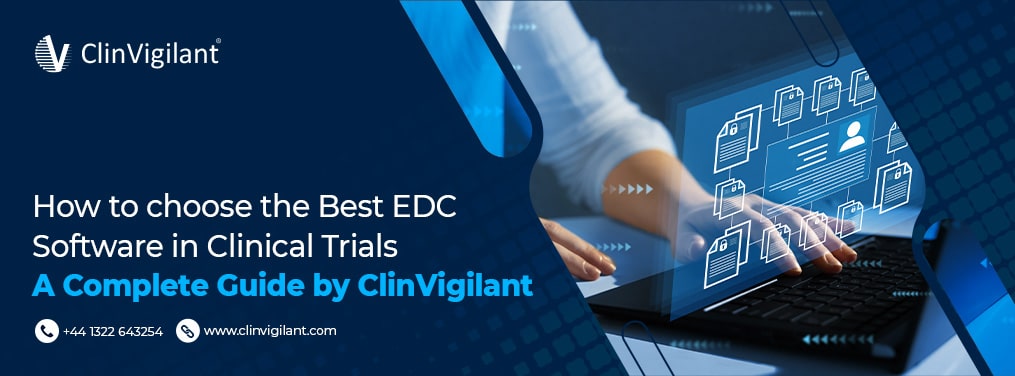
Clinical trials are an essential part of the drug development process. They help determine the safety and efficacy of new treatments and drugs. For clinical trials to be successful, effective data collection, management, and analysis are crucial. However, traditionally, clinical trials have relied on paper-based data capture methods. While paper case report forms (CRFs) were once the norm, they have significant drawbacks that can negatively impact clinical trials.
This has led to the emergence of electronic data capture (EDC) systems and a shift away from paper processes. EDC has brought immense improvements to clinical trial processes, but the integration of artificial intelligence (AI) is poised to further enhance EDC capabilities. This guide will provide a complete overview of EDC in clinical trials, explain how AI integration is transforming EDC, and explore what the future holds for AI-enabled EDC software in clinical research.
What is EDC in Clinical Trials?
Electronic Data Capture Software In clinical trials (EDC) refers to the use of electronic systems to collect and manage clinical trial data. EDC software replaces traditional paper CRFs with electronic case report forms (eCRFs) and online data capture tools. EDC systems may include web-based platforms, mobile apps, wearable devices, and specialized software that allow clinical trial data to be captured electronically from study sites and then stored centrally.
The purpose of EDC is multifold – to streamline data capture, provide real-time access to data, enhance data quality, and facilitate efficient clinical trial conduct. EDC enables sponsors, CROs, and study sites to move away from cumbersome paper processes to streamlined electronic methods for better data oversight.
Key Benefits of EDC in Clinical Trials
The use of EDC delivers immense advantages over paper-based data collection, including:
- Enhanced data quality and integrity: EDC systems allow real-time data validation checks, reducing errors, outliers, and missing data. This results in more complete and reliable data.
- Real-time data capture: Data entered in EDC systems is immediately available for review and analysis. This enables quicker insights and decision-making.
- Integration of electronic source data: Data from medical devices and wearables can feed directly into EDC systems for integrated data capturing.
- Streamlined trial management and efficiency: By automating processes like reporting, EDC allows clinical teams to focus on critical tasks like patient recruitment and engagement.
- Cost and time savings: EDC eliminates the need for paper-based processes, resulting in resource savings of up to 25-30%, per some estimates.
Clearly, EDC brings tremendous enhancements in data quality, trial efficiency, cost savings, and management capabilities compared to paper-based clinical data management.
AI-Enabled EDC Systems in Clinical Trials
Artificial intelligence (AI) integration takes EDC capabilities to the next level. AI-enabled EDC software incorporates technologies like machine learning and natural language processing to further enhance clinical data processes. Some key benefits of AI-powered EDC in Clinical Research Systems include:
- Improved data quality: AI algorithms can identify anomalies in data and raise alerts in real-time to enable corrections. Natural language processing also facilitates better medical data capture.
- Enhanced decision-making: Advanced analytics and machine learning enable quicker insights from data to support better trial decisions.
- Streamlined data management: AI automates processes like categorizing adverse events reported data to optimize data management workflows.
- Real-time monitoring and analysis: Instant access to metrics, analytics, and dashboards support rapid monitoring of site and patient performance.
The Future of AI-Enabled EDC in Clinical Trials
AI-powered EDC is poised to drive significant transformation in clinical trials going forward. Key trends include:
- Integration of cutting-edge technologies: EDC systems integrating wearables, mobile apps, sensors, and AI will enable real-world data capture.
- Real-time patient-generated data: Patients will be able to feed data directly into EDC systems through connected devices and apps.
- Remote monitoring and engagement: Sponsors will be able to monitor patients and sites remotely in real-time through AI-enabled centralized data access.
- Hyper-efficient trial management: End-to-end workflow automation through AI will optimize trial conduct and oversight.
Conclusion
The integration of AI and machine learning has immense potential to enhance EDC software capabilities and transform clinical trial data processes. AI-enabled EDC can drive higher data quality, accelerated insights, optimized decision-making, streamlined workflows, and reduced costs.
As cutting-edge technologies proliferate, AI-powered EDC will be integral to the future of clinical trials. By enabling hyper-efficient trial management and real-world data capture, AI-EDC solutions will be key to unlocking faster and leaner drug development processes.